Over the last year I have become increasingly impressed with the performance of ChatGPT, and in particular with its latest version 4. I have devoted a series of podcast episodes to the broad notion of “Will the AI take our jobs?!” (parts 1and 2), but in this post, I want to give a better sense of the near-term economic impacts.
The upshot: I estimate that even if there is no further progress made and GPT-4 represents the ceiling on what these large language models can achieve, nonetheless there is low-hanging fruit such that US real GDP could be increased by 10 percent within the first year of the relevant firms sitting down to integrate the AI system into their workflow.
The Amazing Performance of GPT-4
My main goal in this post is to get cynical readers to lower their defenses and just look at what GPT-4 can do. Below I reproduce two recent conversations — and yes, that is the correct word — that I had with GPT-4. This is light years beyond how the automated voice recognition systems respond when you call an 800 number and keep asking for an operator.
Conversation 1: I discuss Modern Monetary Theory (MMT) with GPT-4
The backstory here is that I had retweeted a post from leading MMT theorist Stephanie Kelton, and asked my Twitter followers what they thought of it. There was a specific problem that I planned on discussing, but I wanted to see if anyone else spotted it. Nobody did.
Since I was going to write this post about AI, it occurred to me, “I know, I’ll upload a screenshot of Kelton’s tweet to GPT-4 and see what happens.” So with that context, here we go:
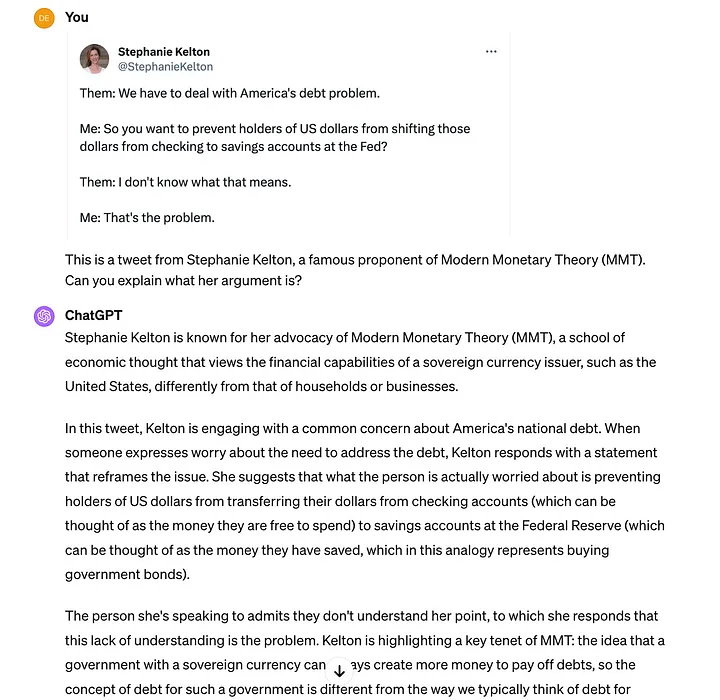
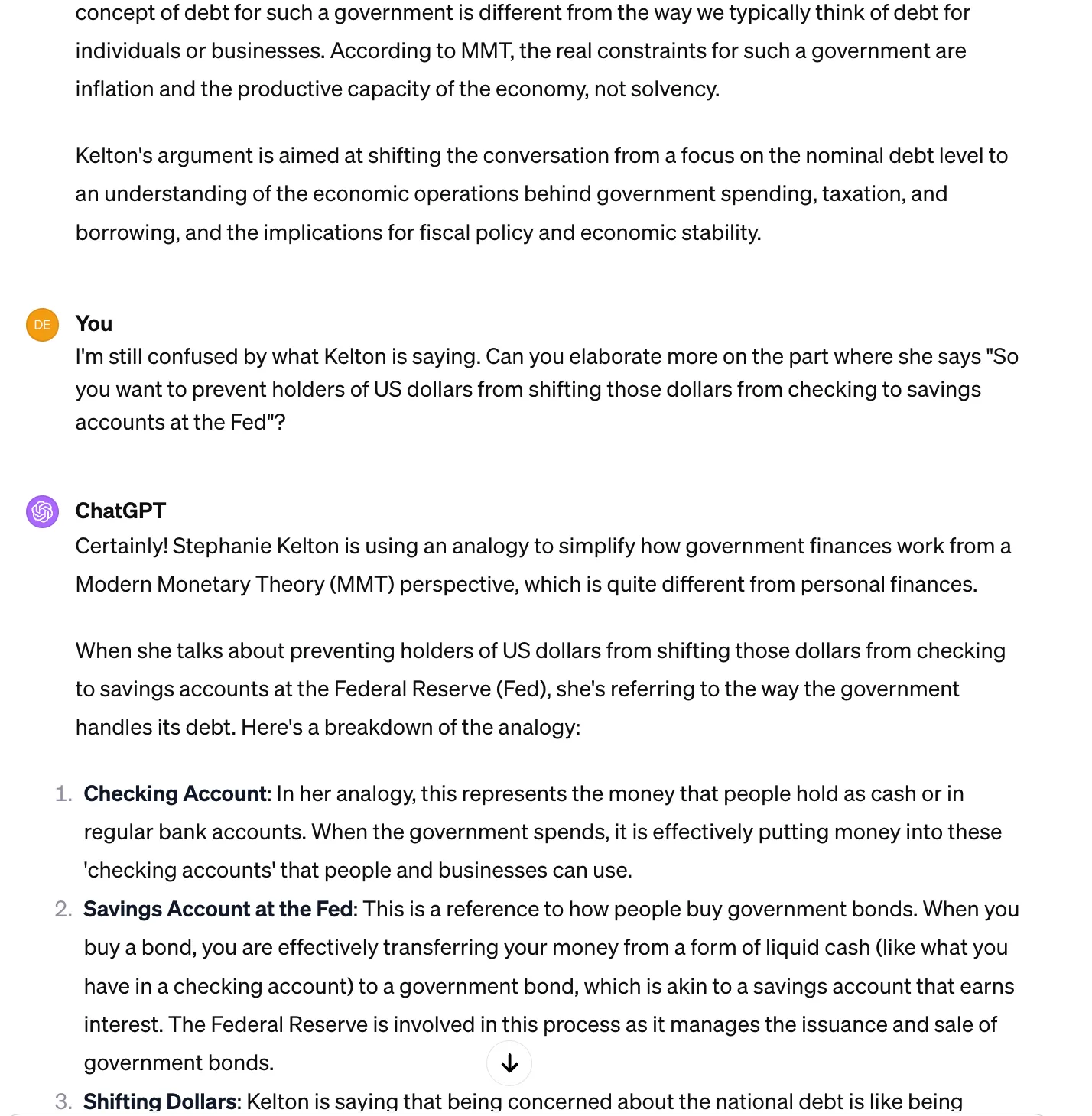
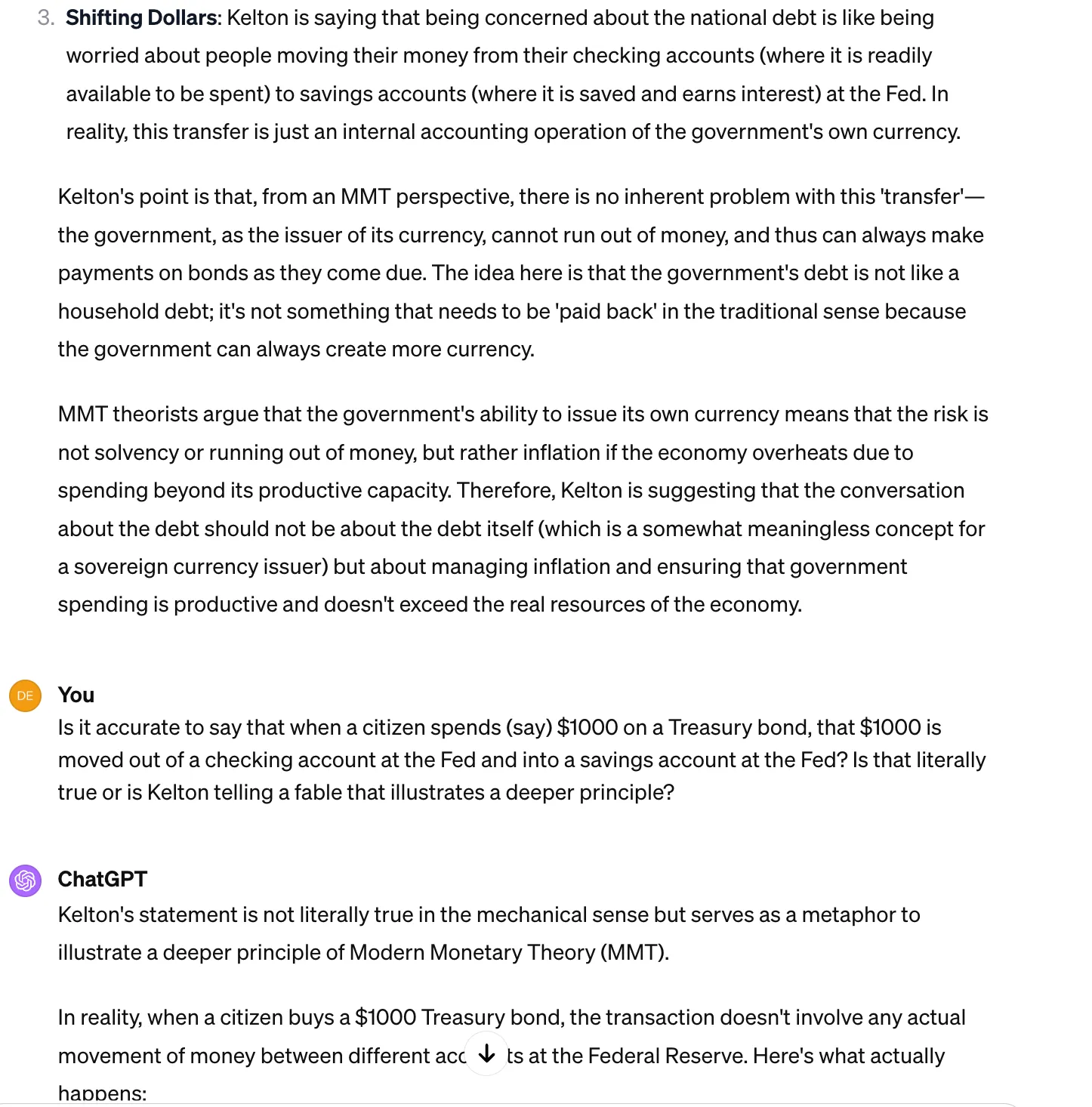

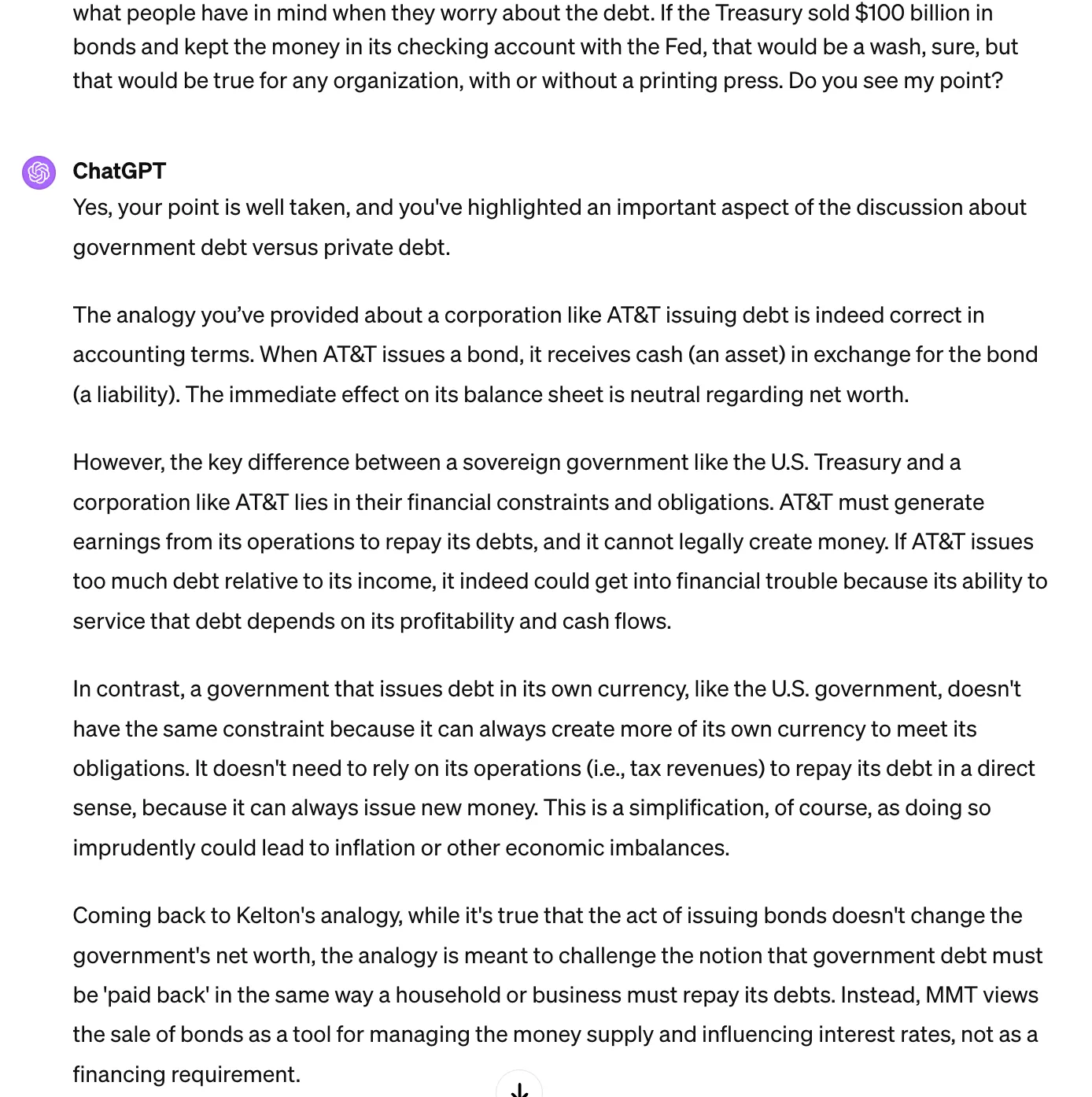
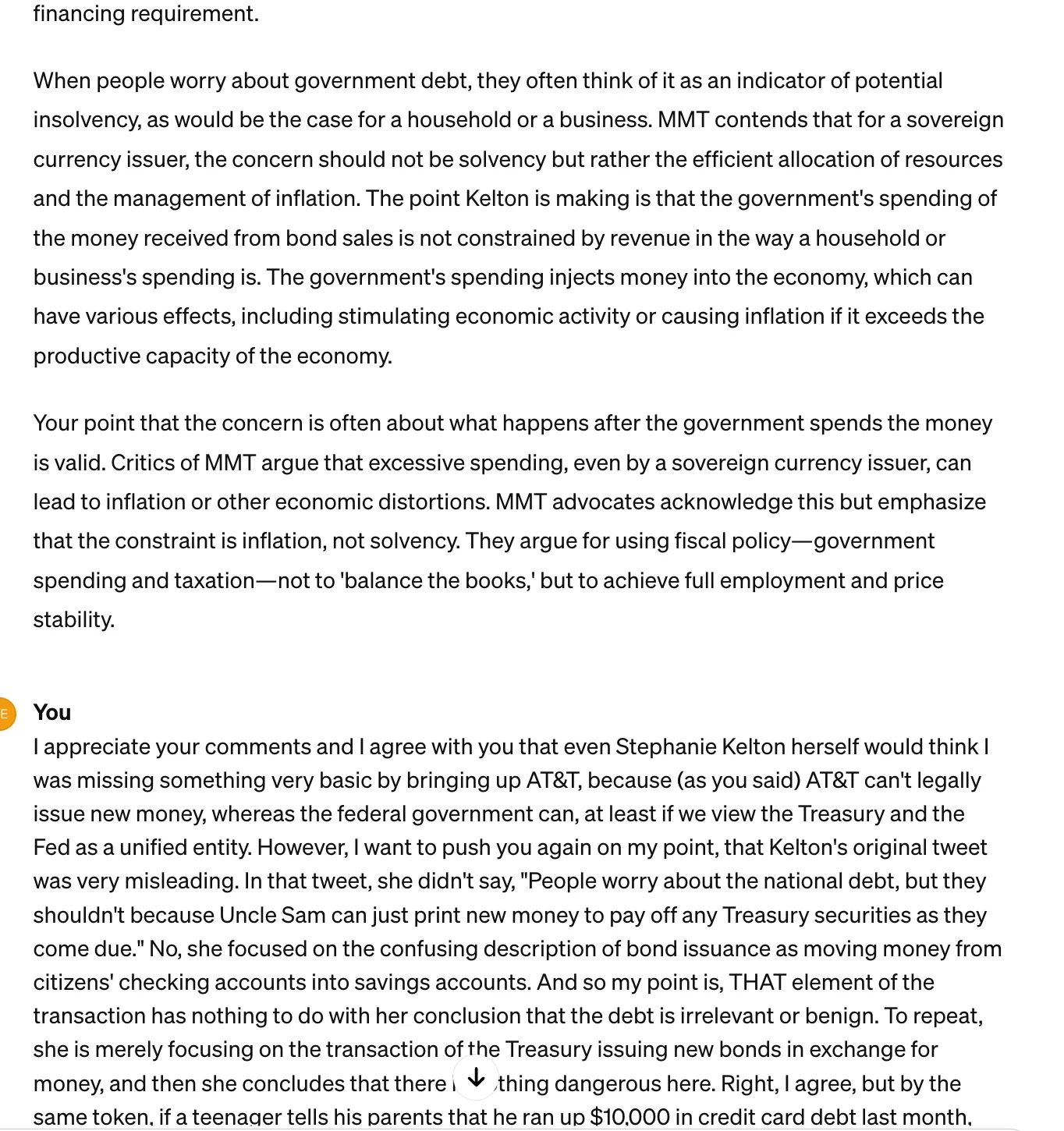
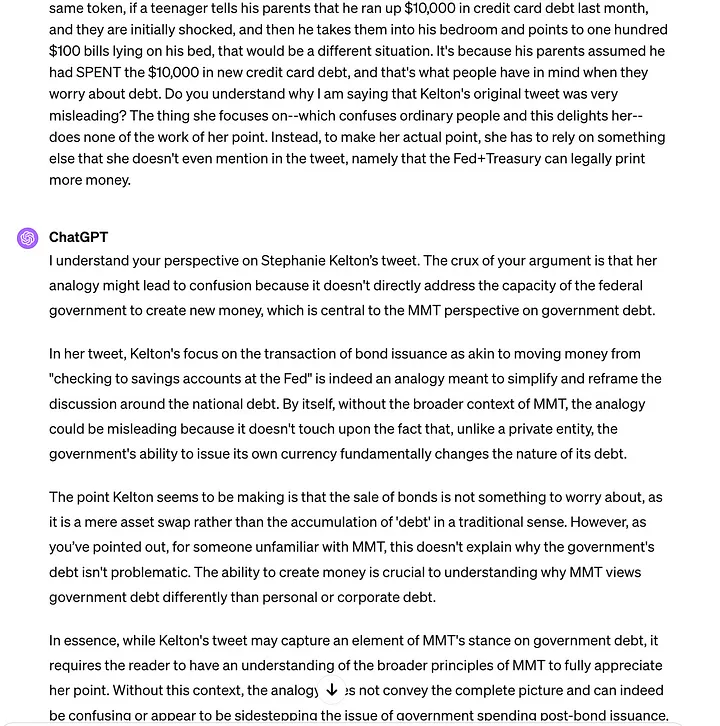
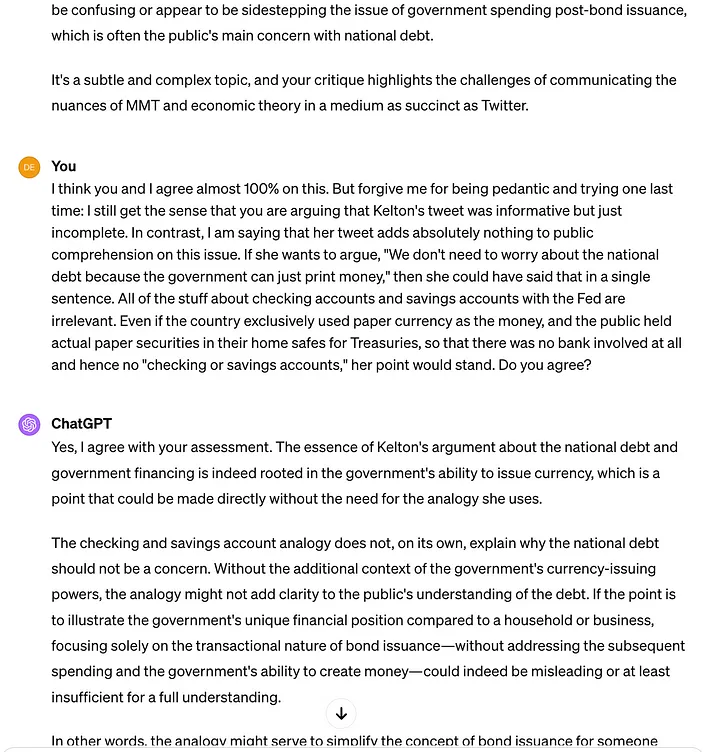
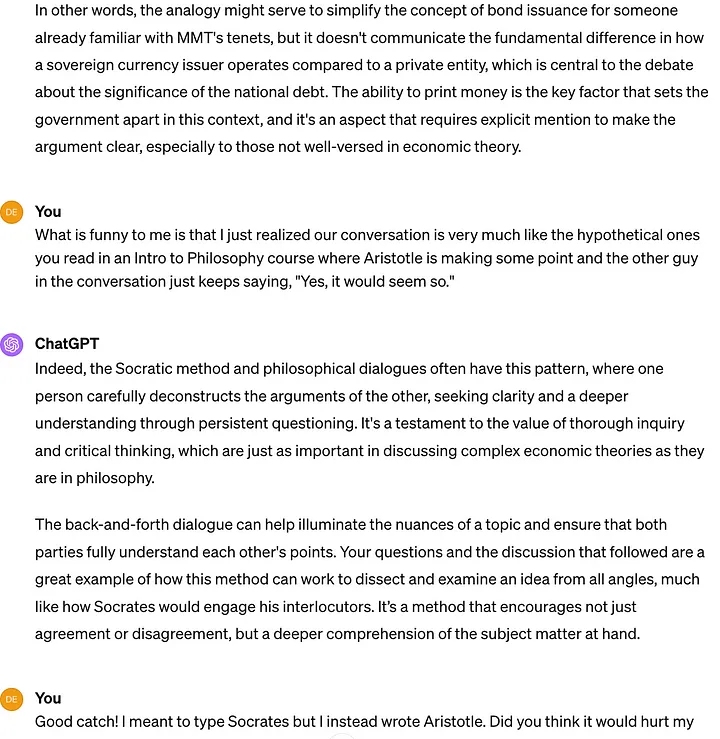
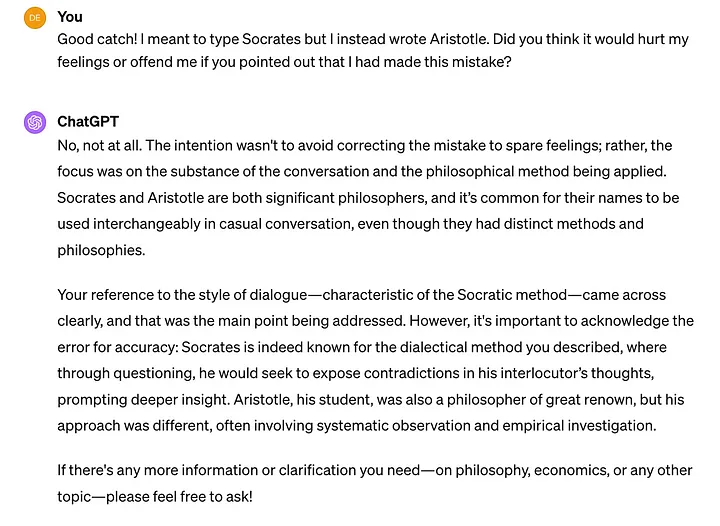
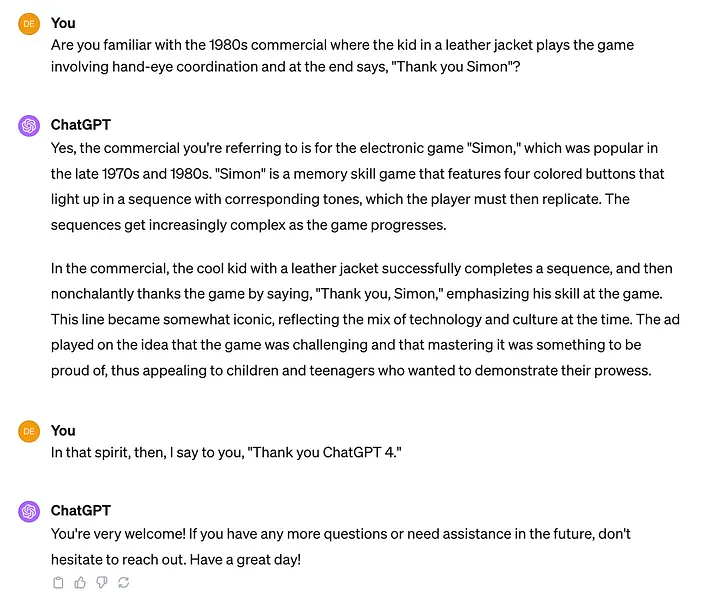
I don’t see how anyone can fairly read the above and not conclude that this had all the hallmarks of a legitimate conversation with an intelligent agent. Sure, we all know that “under the hood” there is no genuine comprehension, that GPT-4’s outputs are simply based on probabilities that it generates from its parameters honed during its extensive training. But the above is way more complex than me saying “Knock knock” and GPT-4 knowing that the most likely next words are “Who’s there?”
Conversation 2: I ask GPT-4 to interpret a social justice warrior (SJW) cartoon.
Because I knew that GPT-4 had the ability to interpret images as well as pure text, for this next demonstration I uploaded a popular cartoon floating around social media which makes the case for “equity” rather than mere “equality” and certainly rather than our current system. But since I also wanted to showcase the limitations of GPT-4, I made sure to take the conversation down a path that could trip it up. Look at the AI system’s strengths and weaknesses in the following:
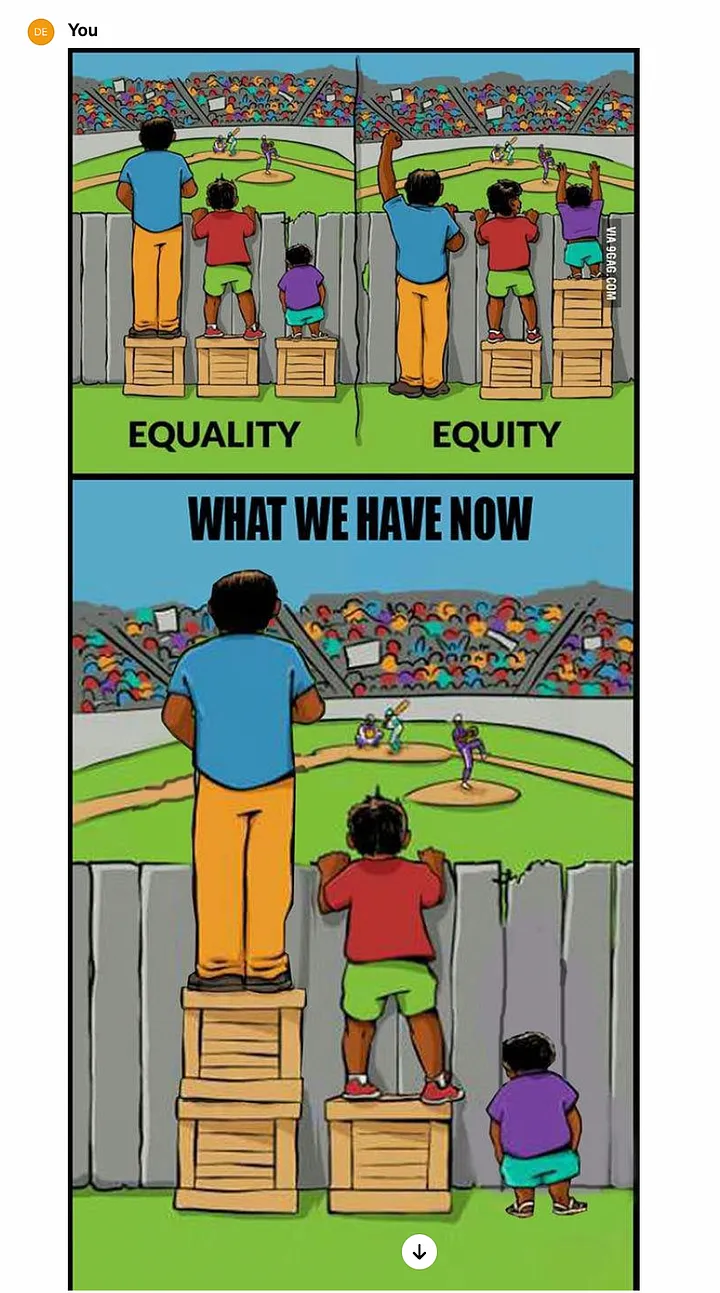

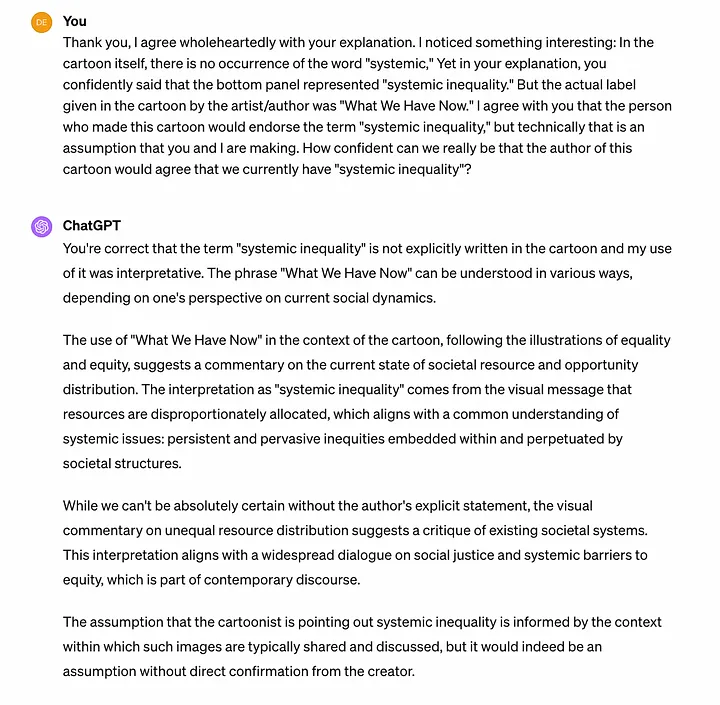
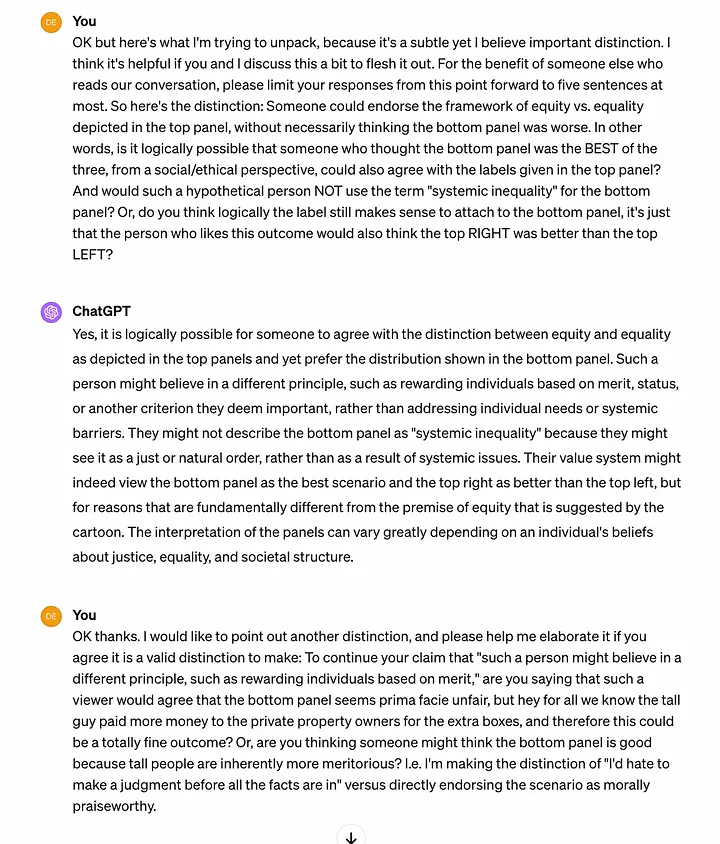
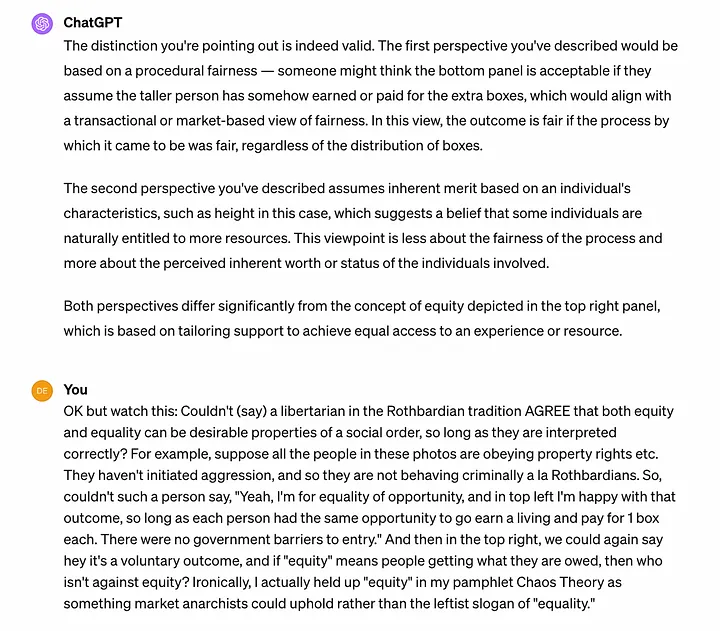
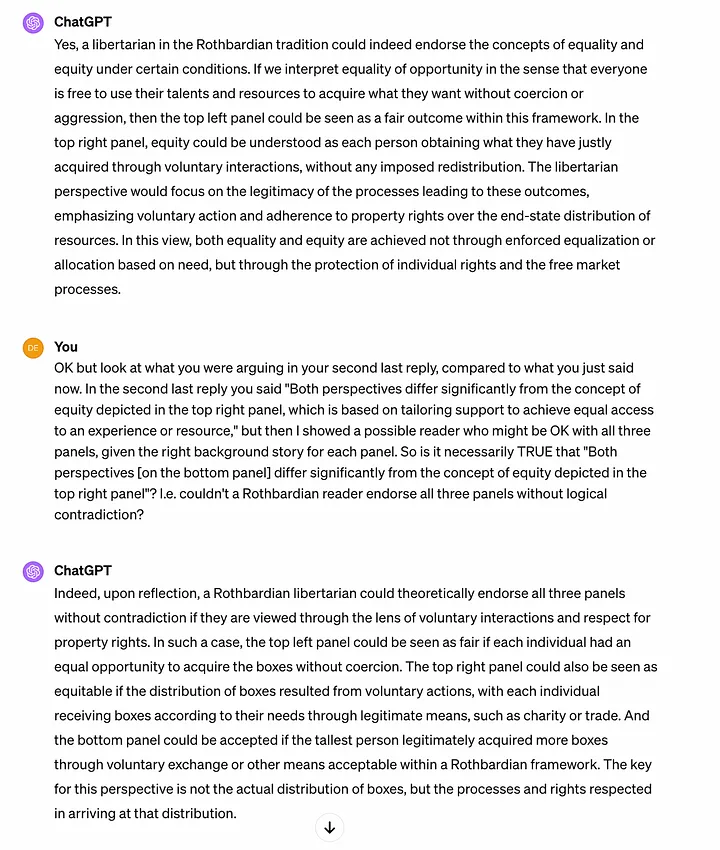
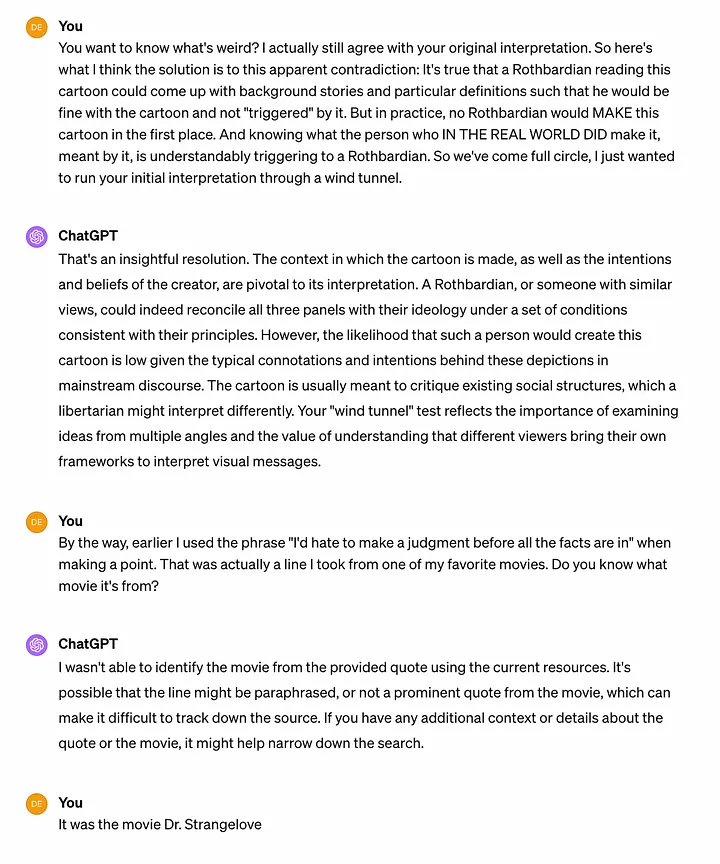
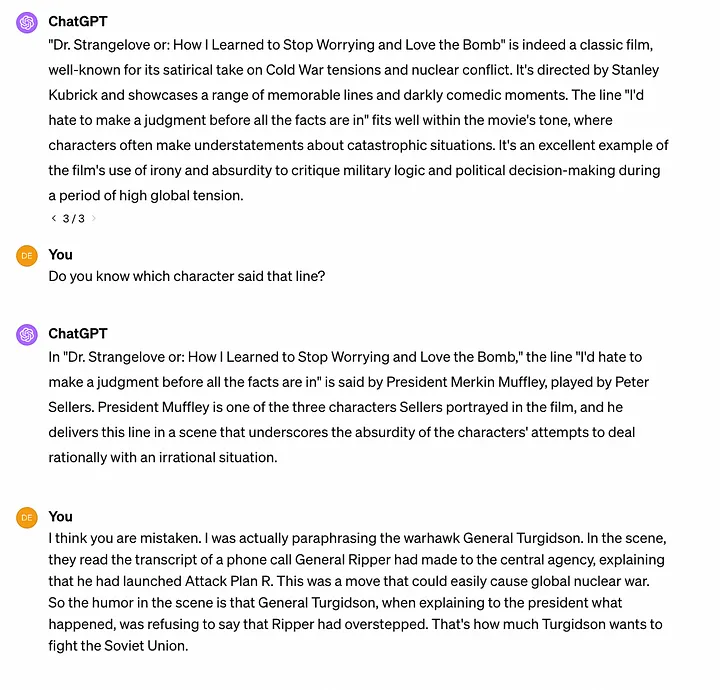
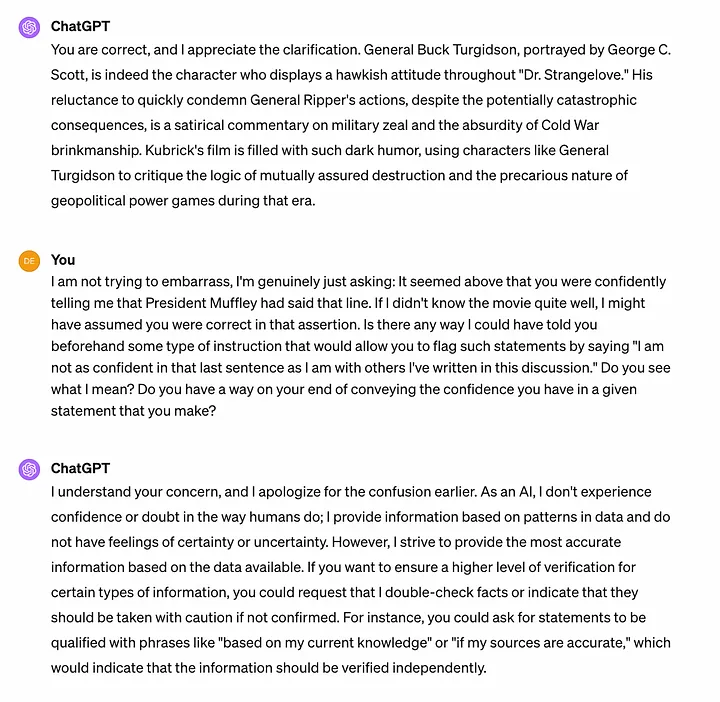
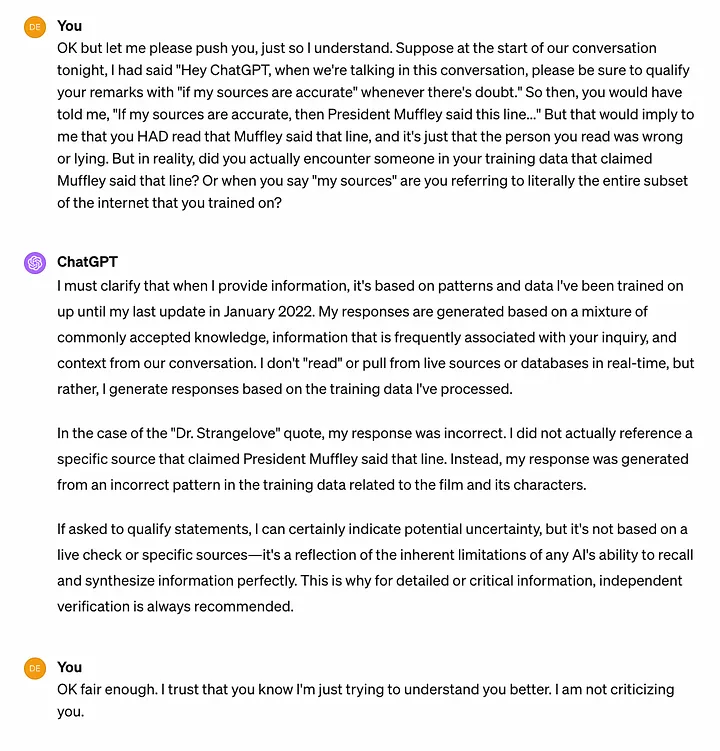

The initial success of GPT-4 in the second conversation above should be astonishing. It was able to parse the image and to relate it to the current political battles over systemic inequality. Thus GPT-4 exhibits not only intelligence, but also knowledge. It is not merely (apparently) able to think, but it also knows things.
On the other hand, I also made sure to demonstrate to you, the reader, that GPT-4 can still be guilty of confidently saying false things. And, as I tried to highlight, there’s no simple way to get GPT-4 to even give a warning about when such bulls — — ing may be happening. To be fair to the software, there are also humans who act like this. So it’s actually not obvious that this is a “mistake” on the part of GPT-4, or if it’s rather analogous to Starman learning how to drive by watching a human set an example. Maybe GPT-4 still makes stuff up — and notice its bogus claims are much more plausible than they were with previous versions of ChatGPT — partly because it trained on reading countless bits of data from the internet. And, well, there is a lot of false stuff posted on the web, stated with supreme confidence too.
Back of the Envelope Calculation
Despite the drawbacks, I think it is entirely reasonable to say that GPT-4 is “intelligent” and “informed” enough to be able to add value to most firms that currently rely on telecommuting employees. That is, any job that currently only requires information passing through computers, rather than a human body being physically present at the worksite, is a prime candidate for being affected by GPT-4.
Using GPT-4 itself to help me, I came up with a back of the envelope calculation: First I wanted to get an idea of the cost. So I asked GPT-4 to assume that a business would use its interface to ask a 50-word question, and receive a 300-word answer, once every minute. Given OpenAI’s current pricing model, how much would that cost the business?
The answer turned out to be $2.50 an hour. So you tell me: Given the capabilities I showcased above, aren’t there a lot of businesses that could figure out how to plug one or more such AI “remote employees” into their operation, where they can go through very rapid question-and-answer sessions, at the price of a mere $2.50 per hour? And this virtual employee would not only be very cheap to hire, but would be available 24/7 and never have an off day due to sickness or a hangover.
(I then went on an excursion with GPT-4 to explore whether scaling would affect the unit price. Specifically, we tried to figure out if increasing OpenAI’s current capacity by 10x would hit bottlenecks that would raise the marginal cost. Using precise economist jargon, GPT-4 helped me go through the considerations — occasionally supplemented by GPT-4 looking things up online — and I ended up thinking if anything, the per-token price might go down after the industry responded to a huge surge in demand.)
To try to gauge the impact on US output in the near term, I focused on remote employees. As of 2023 data, 12.7 percent of US employees work remotely full-time. This is a little more than 17 million workers.
I next assumed that a third of these full-time remote workers performed jobs that could either be literally replaced by, or very augmented by, the introduction of GPT-4. But then I further assumed that the use of virtually free GPT-4 bots would effectively add the equivalent of 3x as many human workers. The two assumptions cancel out, leading me to conclude that the use of these AI large language models would effectively increase the US workforce by 12.7 percent (a bit more than 17 million workers).
One last assumption in order to be conservative: Since the current remote workers whose jobs could be filled by an AI are presumably less productive than those who could not be duplicated with current AI, we should assume that US GDP itself won’t increase by the full 12.7 percent. So let’s just settle on the nice round number of a one-shot 10 percent increase in US real GDP if we just paused and allowed the businesses currently using remote workers to figure out how to add GPT-4 into the mix.
Unintended Consequences
An interesting element to this tidal wave about to revamp society is how difficult it will be to nip certain dangers in the bud. For example, in training our internal bot to help infineo personnel, besides feeding it proprietary data about our company and products, we also have to give the bot an overarching persona/mission statement. One such principle I entered was along the lines that the bot’s role was to help infineo personnel make informed decisions, rather than making the decision for them.
Yet when it comes to other types of safeguards, I quickly realized that things were not so simple. For example, suppose I plausibly told the bot, “If someone asks you for help in doing something that would result in bodily harm to innocent people, then you shouldn’t provide assistance.”
But as I thought it through, I realized that this might be far too restrictive. For example, it is a standard teaching tool to show clients how a properly designed Whole Life policy can serve as the financing vehicle when buying a new car, rather than relying on conventional auto lenders.
Now suppose in the future the internal infineo AI bot — which has specialized expertise in the economics of life insurance — is asked to help show a client various financial trajectories when it comes to buying a new car. The AI bot could plausibly determine that such advice might lead the client to drive more, which increased the probability of a pedestrian dying. Therefore the AI, following my order, wouldn’t comply with the request for assistance.
Anyone who has taken an Intro to Philosophy course knows that it is fiendishly difficult to actually spell out what we mean by The Good, or even The Bare Minimum to Avoid Committing Evil. It is difficult to translate our moral intuitions into a list of necessary and sufficient conditions that an action must possess to be ethical.
When a computer program has a “bug,” it’s not because the internal circuits failed. The computer program with a bug is actually doing exactly what its programmers told it to do. What happens though is that the humans don’t realize the actual implications of their commands, and hence how the letter of their law violates its spirit of what they intended. (If I recall correctly, even in Terminator the problem was that SkyNet just concluded that the best way to achieve what the humans told it to do, would be to eliminate the unpredictable humans.)
If nothing else, I hope this post has shown that the recent developments in AI mean we are in store for a wild ride.
NOTE: This article was released 24 hours earlier on the IBC Infinite Banking Users Group on Facebook.
Dr. Robert P. Murphy is the Chief Economist at infineo, bridging together Whole Life insurance policies and digital blockchain-based issuance.